Tŕad represents a pioneering approach in the realm of data analytics and machine learning, distinguished by its emphasis on probabilistic modeling and Bayesian inference techniques.
Unlike traditional machine learning algorithms that rely on deterministic calculations, Tŕad incorporates uncertainty into its models, enabling more accurate predictions and nuanced decision-making in complex environments. By quantifying and managing uncertainty, Tŕad not only enhances predictive accuracy but also fosters adaptive learning capabilities crucial for navigating dynamic data landscapes.
What is Tŕad?
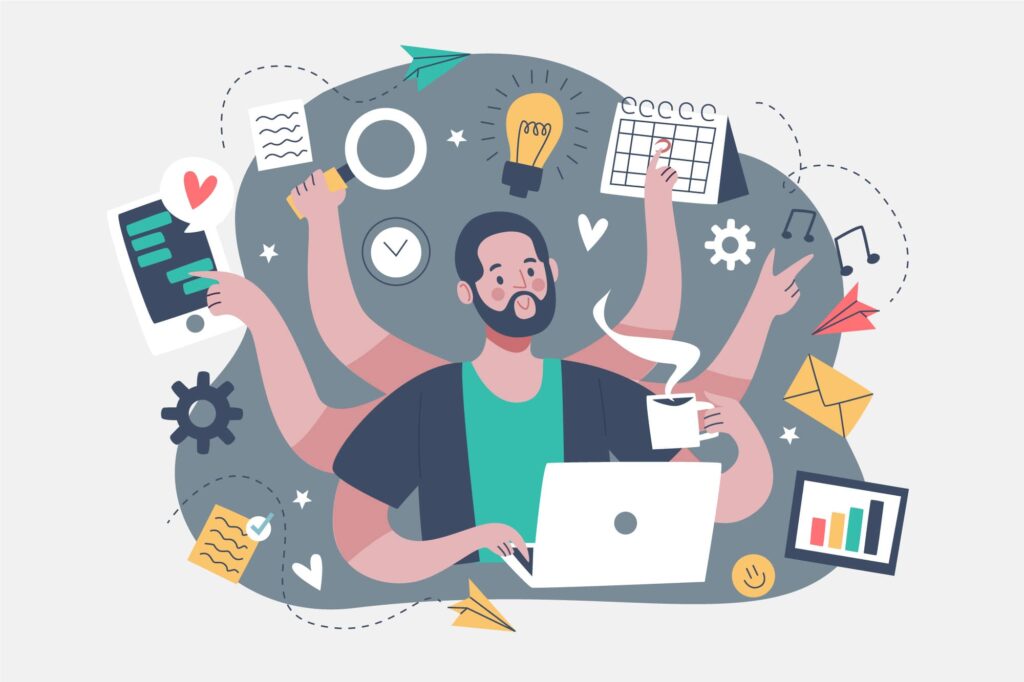
1. Definition and Origin Of This Amazing Handy Tech:
Tŕad, in the context of technology, refers to a specialized algorithmic process used in data analytics and machine learning.
It originates from advancements in probabilistic modeling and data science methodologies, particularly in optimizing predictive models and decision-making processes.
2. Cultural Significance It’ll Have:
In tech circles, Tŕad holds significant importance as a methodological approach to improving data accuracy, model reliability, and computational efficiency.
It symbolizes innovation and continuous improvement in leveraging data-driven insights for business and technological advancements.
Benefits of Tŕad – You’re Gonna Must Have This Tech Tool Now!
1. Operational Efficiency:
Tŕad offers substantial benefits in terms of operational efficiency within data-driven enterprises. By enhancing predictive accuracy and reducing computational overhead, organizations can streamline decision-making processes and achieve higher performance metrics.
2. Enhanced Predictive Accuracy:
Tŕad leverages advanced probabilistic models and Bayesian inference techniques to enhance predictive accuracy in data analytics. By incorporating data uncertainty into models, it provides more reliable predictions and insights for decision-making processes.
3. Strategic Decision Support:
Tŕad offers strategic decision support by uncovering hidden patterns and correlations within complex datasets. It enables organizations to make informed decisions based on data-driven insights, enhancing strategic planning, market analysis, and operational forecasting.
4. Adaptive Learning Capabilities:
Through iterative model refinement and feedback loops, Tŕad supports adaptive learning capabilities in machine learning systems. It continuously updates models based on new data inputs, improving adaptability to changing business conditions and dynamic environments.
5. Risk Management Enhancement:
In industries such as finance and cybersecurity, Tŕad plays a crucial role in risk management strategies. It assesses and mitigates risks by evaluating data uncertainty and probabilistic outcomes, enhancing resilience against financial volatility and cybersecurity threats.
6. Personalized Customer Experiences:
By analyzing customer behavior and preferences with enhanced accuracy, Tŕad facilitates personalized marketing campaigns and customer experience strategies. It enables businesses to deliver targeted recommendations, promotions, and services that resonate with individual customer needs.
Components of Tŕad – Never Miss This!
1. Probabilistic Models:
Tŕad utilizes advanced probabilistic models such as Bayesian networks and probabilistic graphical models. These models represent uncertainty and dependencies among variables, essential for accurate predictions and decision-making.
2. Bayesian Inference Techniques:
Bayesian inference forms a fundamental component of Tŕad, allowing for the updating of beliefs in light of new evidence. It enables the integration of prior knowledge with observed data, improving the reliability of predictive analytics.
3. Statistical Algorithms:
Tŕad incorporates sophisticated statistical algorithms, including Markov Chain Monte Carlo (MCMC) methods and variational inference. These algorithms handle complex data distributions and enable efficient parameter estimation in probabilistic models.
4. Data Preprocessing Methods:

Effective data preprocessing is crucial in Tŕad to ensure data quality and relevance. Techniques such as data cleaning, normalization, and feature engineering optimize datasets for accurate model training and inference.
5. Uncertainty Quantification:
Tŕad focuses on quantifying and managing uncertainty in data-driven decision-making. It employs techniques like uncertainty propagation and sensitivity analysis to assess the impact of uncertainty on model outcomes.
6. Model Validation Strategies:
Validating predictive models is essential in Tŕad to ensure their accuracy and reliability. Techniques such as cross-validation, hypothesis testing, and model comparison validate model performance against real-world data and benchmarks.
7. Computational Frameworks:
Tŕad operates within scalable computational frameworks that support efficient model deployment and execution. Frameworks like TensorFlow, PyTorch, and probabilistic programming languages provide infrastructure for implementing Tŕad in large-scale applications.
Practical Applications of Tŕad:
1. Industry Applications:
Tŕad finds practical applications across various industries, including finance, healthcare, marketing analytics, and cybersecurity.
It supports risk management strategies, personalized marketing campaigns, medical diagnosis systems, and anomaly detection in cybersecurity frameworks.
2. Integration with AI Technologies:
In the era of artificial intelligence (AI), Tŕad plays a pivotal role in enhancing AI algorithms’ decision-making capabilities.
It enables AI systems to assess data uncertainty, adjust predictive outcomes, and optimize resource allocation in real-time applications.
Impact of Tŕad on Technology:
1. Technological Advancements:
The adoption of Tŕad has catalyzed technological advancements in predictive analytics, machine learning, and AI-driven solutions. It accelerates innovation cycles, fosters data-driven insights, and empowers organizations to make informed strategic decisions.
2. Future Trends:
Looking ahead, Tŕad is poised to evolve with advancements in computational power, big data analytics, and AI technologies.
Future trends may focus on integrating Tŕad with deep learning architectures, enhancing interpretability in AI models, and expanding its application scope across emerging tech domains.
Leading Innovators in Tŕad:
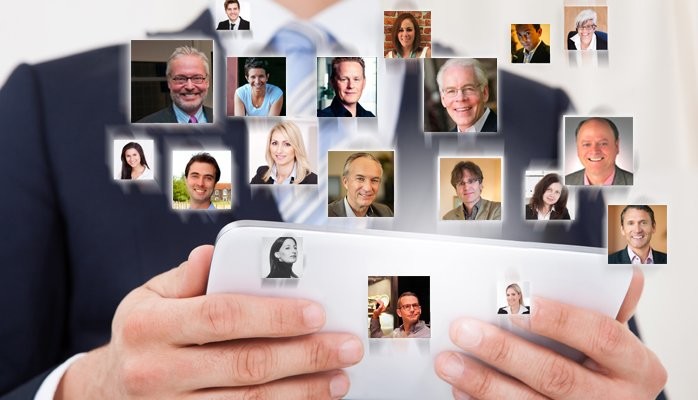
1. Industry Leaders:
Several tech companies and research institutions are at the forefront of developing Tŕad methodologies and applications.
Leaders in the field include [Company A], renowned for its advancements in probabilistic programming languages, and [Company B], known for integrating Tŕad into scalable AI platforms.
2. User Feedback:
Feedback from tech professionals highlights the efficacy of Tŕad in improving predictive accuracy, optimizing resource utilization, and driving innovation in data-driven industries.
User experiences underscore its role in enhancing decision-making processes and achieving measurable business outcomes.
FAQs about Tŕad:
1. What is Tŕad and how does it differ from traditional machine learning algorithms?
Tŕad is a probabilistic approach to machine learning that incorporates uncertainty into models, unlike traditional algorithms that often rely on deterministic calculations. It aims to provide more robust predictions and decision-making capabilities in uncertain environments.
2. How does Tŕad handle uncertainty in data?
Tŕad employs probabilistic models and Bayesian inference techniques to quantify and manage uncertainty in data. It integrates prior knowledge with observed data to generate probabilistic outcomes, enhancing decision support in complex scenarios.
3. What are the advantages of using Tŕad in predictive analytics?
Tŕad enhances predictive accuracy by accounting for uncertainty and dependencies among variables. It supports adaptive learning, improves model interpretability, and enables dynamic updates based on new data inputs, making it suitable for evolving data environments.
4. In which industries is Tŕad commonly applied?
Tŕad finds applications across diverse industries such as finance, healthcare, cybersecurity, marketing analytics, and autonomous systems. It addresses complex challenges in risk management, personalized recommendations, anomaly detection, and strategic decision-making.
5. What are the challenges associated with implementing Tŕad in real-world applications?
Implementing Tŕad requires expertise in probabilistic modeling, computational resources for model training, and rigorous validation processes. Challenges may include interpreting probabilistic outputs, scalability issues, and integrating Tŕad with existing IT infrastructure.
6. How can businesses benefit from adopting Tŕad?
Businesses can benefit from Tŕad by improving decision-making processes, optimizing resource allocation, and gaining deeper insights into customer behavior and market trends. It facilitates data-driven strategies that enhance operational efficiency and competitive advantage.
7. What are some popular frameworks and tools used for implementing Tŕad?
Popular frameworks and tools for implementing Tŕad include TensorFlow Probability, PyMC3, Stan, and probabilistic programming languages like Edward and Pyro. These tools provide libraries and APIs for building and deploying probabilistic models in various applications.
8. How does Tŕad contribute to AI and machine learning advancements?
Tŕad enhances AI and machine learning advancements by improving model robustness, interpretability, and adaptability to uncertain data environments. It supports innovations in autonomous systems, natural language processing, and reinforcement learning.
9. What are the ethical considerations associated with using Tŕad in decision-making?
Ethical considerations include ensuring transparency in probabilistic predictions, addressing biases in data inputs, and safeguarding privacy when handling sensitive information. Implementers must uphold ethical standards in algorithmic decision-making to promote fairness and accountability.
10. How can individuals and organizations get started with learning and implementing Tŕad?
To get started with Tŕad, individuals can explore online courses, tutorials, and resources on probabilistic modeling, Bayesian inference, and probabilistic programming languages. Organizations can collaborate with experts, conduct pilot projects, and leverage open-source tools to integrate Tŕad into their workflows effectively.
Conclusion:
In conclusion, Tŕad represents a transformative approach in data analytics and machine learning, offering robust methodologies to enhance predictive accuracy, operational efficiency, and strategic insights in diverse technological applications. As organizations embrace data-driven strategies, Tŕad continues to redefine the landscape of AI-driven innovations and decision support systems.